1. Choose the learning tool
Plug-in version:
a. Open Praat and click on the menu Praat -> PENTAtrainer2 -> Learn
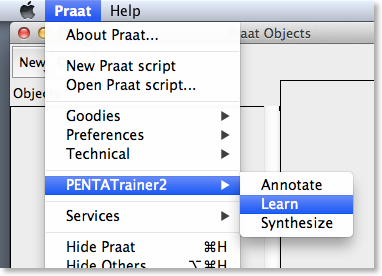
b. Choose the working folder. Note that this folder me has already been annotated by the PENTAtrainer2's Annotation tool to generate necessary data files for optimization.
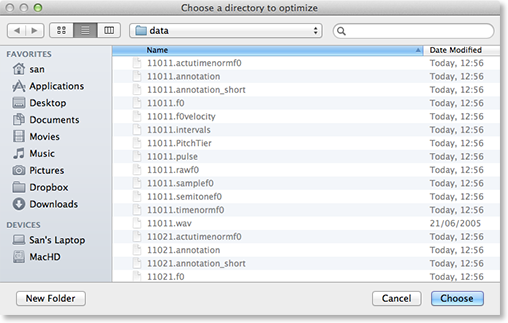
Script version:
Select "Learn" from the drop-down menu
3. Input the optimization parameters. Each parameter control the behavior the learning process, as described below. Click Start to begin.
4. After clicking Start, the optimization window will pop up with the progress bar indicating the progress of the optimization process.
5. Once completed, the optimization window will display the error and correlation information. These measurements were taken by comparing the synthetic with the original F0 contours. Click Close to finish the optimization process.
6. If users select Inspect Manipulation, Praat Manipulation object of each sound file integrating with the synthetic F0 contour (green dots) will be generated. Users can visually inspect and listen to the synthesized sound by inspecting this object.
7. The optimized parameter values are stored in the “parameters.txt” file. Users can use either text editing or spreadsheet programs to open the file.
8. Using a spreadsheet program (e.g., Excel), users can sort the parameters to make them easier to understand. In this example, sort the parameter by “Focus” then “Tone” and then “Sentence”. The figure shows part of the sorted parameters. A number of interesting observations can be made from this data. It shows that F (Falling) tone has negative slope while R (rising) tone has positive slope. H has almost static slope with a relatively high target height while L has much lower target height. Pitch targets of LS (sandhi L tone) are similar to R.
9. Utterance specific RMSE and correlation of optimized parameters are stored in the “accuracy_learning.txt” file.
10. The closeness of fit between the original and synthesized F0 contours can also be visually inspected by opening the file [filename].synf0.
11. The changes in the learning errors over iterations can be found in the “total_error.txt” file. Using a spreadsheet program, users can plot the changes in learning errors over iterations.