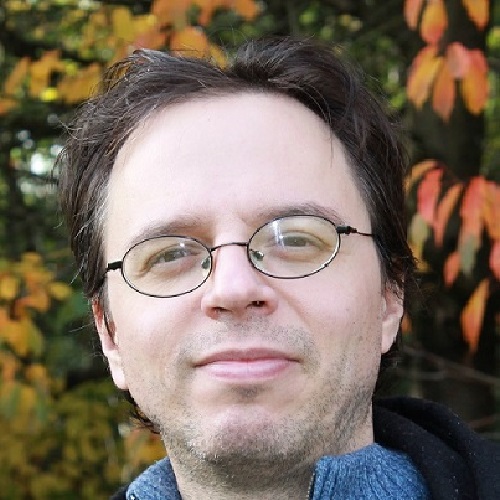
Federico Galvanin
Federico Galvanin received the degree in Chemical Engineering in 2006 and the Ph.D. degree in 2010 at the University of Padova. After a post-doctoral activity in Padova focusing on the optimal design of clinical tests for the identification of physiological models, he has been working as a post-doc Research Associate at University College of London (UK) since 2014.
In September 2015 he started a new position as a Lecturer at the UCL Department of Chemical Engineering, where he became Associate Professor in 2021. His main areas of expertise are in the field of model identification including optimal design of experiments (theory and applications) for the development of chemical, biological and physiological models. In particular, his research activity is related to i) the development of advanced techniques for the optimal model-based design of experiments (MBDoE) in dynamic systems; ii) the application of conventional and advanced design of experiments techniques to physiological systems as well as to chemical processes; iii) the development and online application of model identification techniques in automated systems.
In the group, a systems-based approach is employed for the design of optimally informative experiments with the goal of identifying dynamic models with the minimum cost, time and effort in the experimentation.
Arun Pankajakshan
Arun earned his Bachelor’s degree in Chemical Engineering from TKM College of Engineering, India in 2013. He has received his Master’s degree in Chemical Engineering from Indian Institute of Technology Roorkee, India in 2015. He is currently doing his PhD under the supervision of Dr Federico Galvanin at University College London where he has become a part of the system identification group in the Dept. of Chemical Engineering since January 2017. His research project is focused on the development of a software platform in Python programming language for the online identification of kinetic models in fully automated microreactor systems. The goal of the project is to build robust and numerically stable modelling framework to make the model identification procedure fully automated.
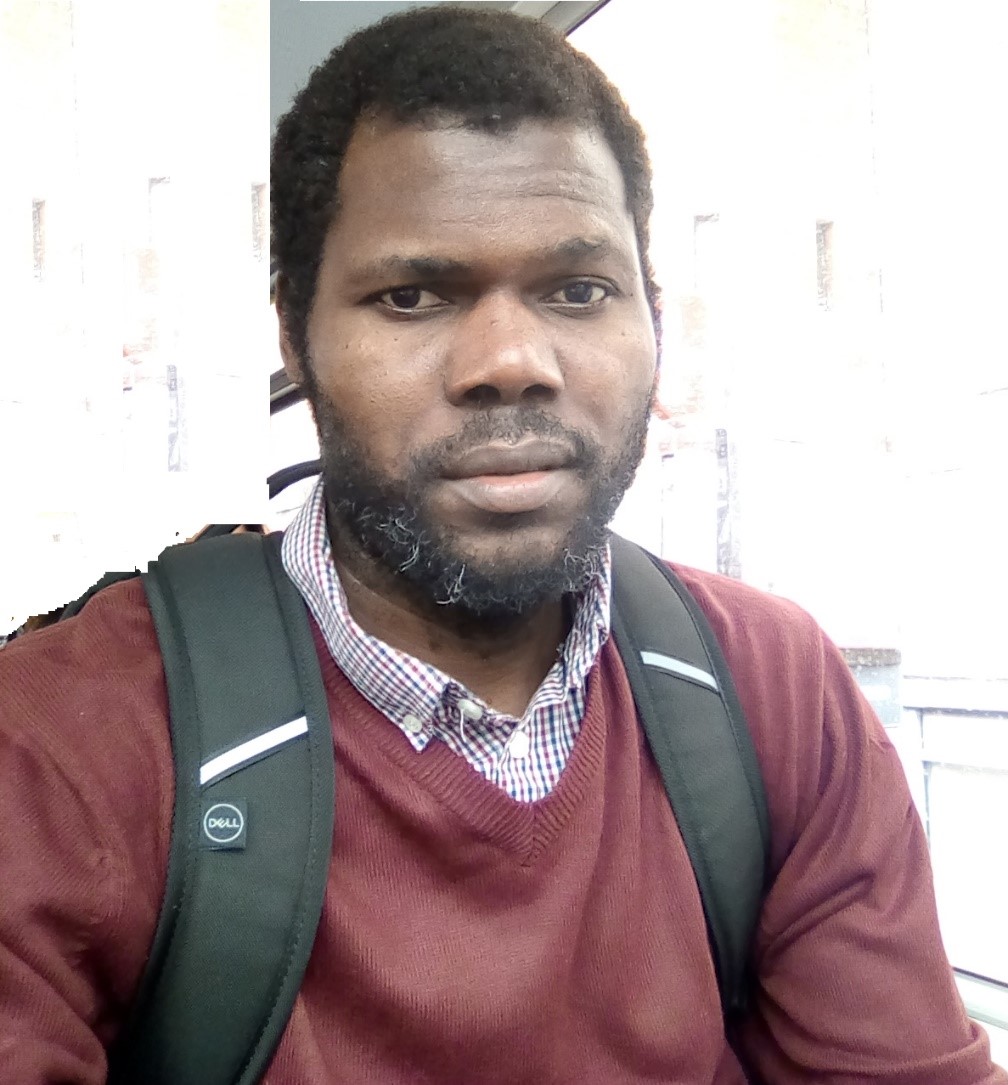
Emmanuel Agunloye
Emmanuel in 2019 obtained the PhD in chemical engineering from University College London, where he developed and investigated models for the synthesis of gold nanoparticles, combining multiphase modelling of population balance for the solid particle phase and continuum material balance for the liquid phase with closure expressions for reaction kinetics and thermodynamics, nucleation, growth, and aggregation. Before joining the Galvanin System Identification Group, he lectured at the University of Lagos, Nigeria, teaching courses in chemical thermodynamics, industrial chemistry, and plant design, and researching in modelling activities covering the Nigerian covid-19 pandemic and industrial chemical production. Fascinated by model-based design of experiments (MBDoE) techniques, Emmanuel employs physics-based models to explore the design space by identifying a minimum set of experiments that would generate the most relevant and informative data from reaction processes. MBDoE involving model development, parameter estimation and model improvement can generate informative experimental data for the development of digital twin models to be used in chemical manufacturing when applied to automated flow reactors scheduled to operate in parallel.
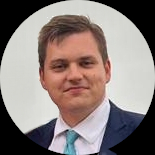
Philipp Deussen
Philipp began studying chemical engineering at UCL in 2015 and has been working in the System Identification Group since 2018 as part of his master’s degree. The thesis focused on finding optimal experimental conditions using the model-based design of experiment technique in order to precisely estimate kinetic parameters in HMF hydrogenation reactions. After completing the MEng degree in 2019, he continued working on the HMF Hydrogenation project, which resulted in the publication of two peer-reviewed papers.Philipp then worked in a mergers and acquisitions company in Germany, with an economics think tank in the UK and studied courses on geology and finance at the University of Basel in Switzerland, before returning to the Systems Identification Group to begin his doctoral studies in 2022. The focus of his current research is to explore the applications of model-based design of experiments frameworks to optimise exploratory drilling patterns in the metals mining industry. The goal is to better identify model parameters and discriminate between models of subsurface systems, in order to increase the efficiency of the exploration process. His research interests also include the development and application of machine learning models to such geological systems.
Andrea Friso
Andrea Friso received his Bachelor's and Master's degree in Chemical and Process Engineering from the University of Padua in February 2022. During his Master's degree, he spent 6 months at UCL as an Erasmus trainee writing his Master's thesis on the simultaneous identification of structure and parameters of kinetic models by coupling Model-Based Design of Experiments (MBDoE) techniques with Machine Learning (ML) techniques, in particular Gaussian processes. In October 2021, he joined the Department of Chemical Engineering at UCL as a Research Assistant and worked on the EPSRC-funded Cognitive Chemical Manufacturing project in collaboration with University of Leeds, AstraZeneca and IBM. Since May 2022 he has been a GSK-funded PhD student in the System Identification Group. His research focuses on modelling and optimisation of autoinjectors for drug delivery systems using MBDoE and ML techniques.
Wenyao Lyu
Wenyao obtained her Bachelor’s degree in Chemical Engineering & Technology from Sichuan University (China) in 2020. She then started her Master’s course in Advanced Chemical Engineering with Process Systems Engineering at Imperial College London and was awarded a distinction grade in 2021. Her Master’s thesis focused on modelling and optimising the heating-CIP cycle in milk pasteurisation using dynamic modelling (gPROMS) and economic & environmental evaluation under the supervision of Prof Sandro Macchietto. She is currently doing her PhD under the supervision of Dr Federico Galvanin at University College London, where she is focusing on developing a new framework based on the integration of physics-informed machine learning (ML) techniques and model-based design of experiments (MBDoE) for the fast identification of kinetic models.
Enrico Sangoi
Enrico obtained both his Bachelor (2018) and Master’s (2020) degree in Chemical Engineering from University of Padova, Italy. He studied at UCL as an affiliate student within the Erasmus+ exchange program during his last year of Master’s, when he also joined the System Identification Group for conducting research on a Machine Learning-based approach to Design of Experiments, which he wrote his Master’s Thesis about. After spending two years in industry, in September 2022 Enrico returned to UCL pursuing a PhD in Chemical Engineering. His research interest is in applying Machine Learning tools to system identification, with special focus about optimal design of experiments applications. He is conducting a research project on using Model-based and Machine Learning-based design of experiments for modelling agrochemical systems, in collaboration with Syngenta.
Ahmad Nabil Eblagh
Ahmad embarked on his academic journey at UCL in 2019, pursuing an MEng degree in Chemical Engineering. After four successful years at UCL, he achieved his master's degree in Chemical Engineering in September 2023. His thesis, supervised by Dr. George Manos, delved into experimental studies on the catalytic pyrolysis of plastic waste. During a summer studentship under the guidance of Dr. Federico Galvanin, Ahmad developed a profound interest in Model-Based Design of Experiments (MBDoE). Motivated by this newfound interest, he seized the opportunity to join the systems identification group and commenced a PhD, funded by Casale SA, in November 2023. The focus of his research involves the creation of an integrated hardware-software platform for the validation of kinetic models and optimization of reaction systems.
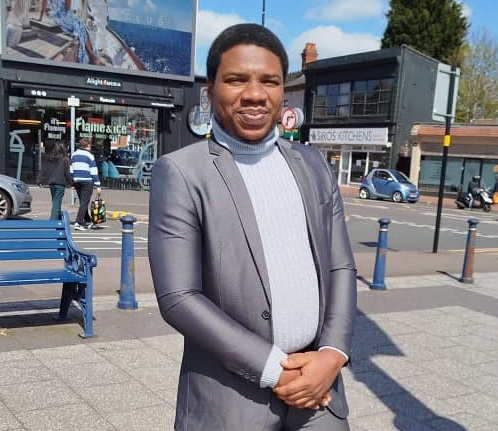
Michael Aku
Michael Aku is a graduate of Chemical Engineering from Ladoke Akintola University of Technology, Ogbomoso, Nigeria, whose passion for technological innovation, software development and AI is remarkable. During his undergraduate days, Michael developed programming skills by writing heavy-ended applications in Matlab, C#, and SQL. He further developed an image processing application using Artificial Neural Network (ANN) and K-Nearest Neighbour (KNN) algorithms. He obtained a scholarship from the Total IFP School France to pursue a Masters’ Degree in Petroleum Engineering where he graduated with distinction. Michael has worked with service companies in the oil and gas industry for a period spanning seven years, gaining experience in building Machine Learning (ML) models by integrating ML-driven applications in the oil and gas industry for hydrocarbon accounting, predictive maintenance and HSE compliance. Due to his outstanding contribution to the industry, he won the SPE African Regional Award on Data Science and Engineering Analytics, where he leads SPE Africa on AI, ML and their applicability in the oil and gas industry. His experience in building ML algorithms, has seen him publishing papers and serving as a mentor for recognised institutes (Tony Elumelu Foundation, Founders Institute). He is currently pursuing a PhD at UCL in the GSIG, where he intends to build a framework for the identification of machine learning models suitable for reaction systems.
Yuxuan Yang
Yuxuan completed his Bachelor's degree in Applied Chemistry at Xi'an Jiaotong-Liverpool University in Suzhou, China, in 2022. Transitioning to the field of engineering, he pursued an MSc in Chemical Process Engineering at University College London after graduation. Under the supervision of Dr Federico Galvanin, his thesis focused on applying Model-Based Design of Experiments (MBDoE) to optimize drug delivery tests for Von Willebrand Disease (VWD), a blood coagulation disorder. Following his completion of the MSc in 2023, he spent nine months in China working in concrete manufacturing. In the summer of 2024, he returned to UCL as a PhD student in the System Identification Group, and his current research focuses on the application of MBDoE to capacitive deionization technology for seawater desalination and water treatment.
Kendall Cui
Jinwen Cui joined University College London in September 2021, and she will obtain her BEng degree in Chemical Engineering from UCL in June 2024. She is currently working on the project "Optimal Design of experiments for identification of catalyst deactivation in flow reactor system" under the supervision of Dr Federico Galvanin. The project involves dynamic modelling in gPROMS ModelBuilder and kinetic model identification using model-based design of experiments (MBDoE) techniques.